Randomized Experiments
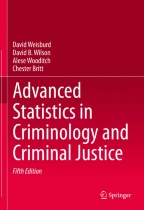
Randomized experiments have become a key method for identifying the effects of treatments or programs on outcomes in criminology and criminal justice. They have also become an important method for identifying how people’s perceptions and attitudes change in different scenarios that are created in the laboratory. We begin the chapter by describing the structure of a randomized experiment and then illustrate why randomized experiments provide a very strong ability to make causal inferences without concern for confounding. We then turn to selected design types and associated analyses. We pay particular attention to block randomized studies and illustrate how they help the reader to maximize equivalence and statistical power in randomized experiments. Finally, we discuss the approach of using covariates in experimental studies as a method of increasing statistical power.
This is a preview of subscription content, log in via an institution to check access.
Access this chapter
Subscribe and save
Springer+ Basic
€32.70 /Month
- Get 10 units per month
- Download Article/Chapter or eBook
- 1 Unit = 1 Article or 1 Chapter
- Cancel anytime
Buy Now
Price includes VAT (France)
eBook EUR 67.40 Price includes VAT (France)
Softcover Book EUR 84.39 Price includes VAT (France)
Hardcover Book EUR 116.04 Price includes VAT (France)
Tax calculation will be finalised at checkout
Purchases are for personal use only
Notes
Statistically, the groups are equivalent in the sense that the expected value of the mean for any baseline characteristics is the same across conditions. However, the observed groups will differ, but these differences will conform to known probability distributions, enabling us to differential between outcome differences that were plausibly due to these random differences or likely due to the experimental manipulation (e.g., treatment).
See Boruch (1997). Econometrics includes other methods, such as instrumental variable analysis, that allow for an unbiased estimation of a treatment effect. These are beyond the scope of this text. However, these methods often rely on naturally occurring random processes, thus mimicking what is discussed here.
See Weisburd and Gill (2014). Stata programs were developed to run a randomization sequence (blocked or naïve) on the JCE dataset and then run a t-test comparing the treatment and control group means at baseline on the three outcomes of interest. Stata’s simulation function was then used to run each program 10,000 times and create a dataset containing the group means, t-values, p-values, an indicator showing whether or not the two groups were significantly different at baseline for each iteration, and the absolute average mean group difference across all iterations.
Of course, this is about what we would have expected given a .10 significance threshold and a fair randomization procedure. But the important point is that the block randomization approach allows us to do better.
In factorial experimental designs, it is ideal to have fully balanced designs. This both simplifies the analysis, as explained below, and maximizes statistical power given a fixed sample size. For block randomized designs, the sample sizes across the levels of the blocking factor are typically unequal. However, it is ideal to ensure balance on the experimental or treatment factor within each level of the blocking factor. That is, block randomized designs are ideally at least partially balanced.
For a factorial experiment where two (or more) factors are manipulated, a Type III model will usually be preferred. In this situation, the unbalanced nature of the design is merely an experimental artifact and should be small in magnitude. Thus, any difference in the sample sizes across cells is random, and giving each cell equal weight in the analysis makes the most sense. That is, conceptually, we are interested in the effects that would be estimated if the design were balanced. However, if the main effects for a Type II versus Type III ANOVA differ, it is wise to explore why that is the case and carefully assess which makes most conceptual sense for your research question.
References
- Ariel, B., Sherman, L. W., & Newton, M. (2020). Testing hot-spots police patrols against no-treatment controls: Temporal and spatial deterrence effects in the London Underground experiment. Criminology, 58, 101–128. https://doi.org/10.1111/1745-9125.12231. ArticleGoogle Scholar
- Boruch, R., May, H., Turner, H., Lavenberg, J., Petrosino, A., De Moya, D., et al. (2004). Estimating the effects of interventions that are deployed in many places: Place-randomized trials. American Behavioral Scientist, 47(5), 608–633. ArticleGoogle Scholar
- Boruch, R., Snyder, B., & DeMoya, D. (2000). The importance of randomized field trials. Crime & Delinquency, 46(2), 156–180. ArticleGoogle Scholar
- Boruch, R. F. (1997). Randomized experiments for planning and evaluation: A practical guide (Vol. 44). Thousand Oaks, CA: Sage. BookGoogle Scholar
- Braga, A. A., Weisburd, D. L., Waring, E. J., Mazerolle, L. G., Spelman, W., & Gajewski, F. (1999). Problem-oriented policing in violent crime places: A randomized controlled experiment. Criminology, 37(3), 541–580. ArticleGoogle Scholar
- Campbell, D. T., & Boruch, R. F. (1975). Making the case for randomized assignment to treatments by considering the alternatives: Six ways in which quasi-experimental evaluations in compensatory education tend to underestimate effects. In C. A. Bennett & A. A. Lumsdaine (Eds.), Evaluation and experiment: Some critical issues in assessing social programs (pp. 195–296). New York, NY: Academic Press. ChapterGoogle Scholar
- Cook, T. D., Campbell, D. T., & Day, A. (1979). Quasi-experimentation: Design & analysis issues for field settings (p. 351). Boston, MA: Houghton Mifflin. Google Scholar
- De Leon, G., Melnick, G., Thomas, G., Kressel, D., & Wexler, H. K. (2000). Motivation for treatment in a prison-based therapeutic community. The American Journal of Drug and Alcohol Abuse, 26(1), 33–46. ArticleGoogle Scholar
- Farrington, D. P. (1983). Randomized experiments on crime and justice. Crime and Justice, 4, 257–308. ArticleGoogle Scholar
- Feder, L., Jolin, A., & Feyerherm, W. (2000). Lessons from two randomized experiments in criminal justice settings. Crime & Delinquency, 46(3), 380–400. ArticleGoogle Scholar
- Flay, B. R., & Best, J. A. (1982). Overcoming design problems in evaluating health behavior programs. Evaluation & the Health Professions, 5(1), 43–69. ArticleGoogle Scholar
- Kirk, R. E. (2013). Research strategies and the control of nuisance variables. In Experimental design: Procedures for the behavioral sciences (pp. 1–30). Thousand Oaks, CA: Sage. ChapterGoogle Scholar
- Lipsey, M. W., Wilson, D. B., Cohen, M. A., & Derzon, J. H. (2002). Is there a causal relationship between alcohol use and violence? In Recent developments in alcoholism (pp. 245–282). Boston, MA: Springer. ChapterGoogle Scholar
- Maxwell, S. E., Delaney, H. D., & Kelley, K. (2017). Designing experiments and analyzing data: A model comparison perspective. London: Routledge. BookGoogle Scholar
- McCord, J. (2003). Cures that harm: Unanticipated outcomes of crime prevention programs. The Annals of the American Academy of Political and Social Science, 587(1), 16–30. ArticleGoogle Scholar
- Meissner, C. A., Redlich, A. D., Michael, S. W., Evans, J. R., Camilletti, C. R., Bhatt, S., & Brandon, S. (2014). Accusatorial and information-gathering interrogation methods and their effects on true and false confessions: A meta-analytic review. Journal of Experimental Criminology, 10(4), 459–486. ArticleGoogle Scholar
- Powers, E., & Witmer, H. (1951). An experiment in the prevention of delinquency. In The Cambridge-Somerville Youth Study. New York, NY: Columbia University Press. Google Scholar
- Redlich, A. D., Quas, J. A., & Ghetti, S. (2008). Perceptions of children during a police interrogation: Guilt, confessions, and interview fairness. Psychology, Crime & Law, 14(3), 201–223. ArticleGoogle Scholar
- Rosenthal, R. (1965). The volunteer subject. Human Relations, 18(4), 389–406. ArticleGoogle Scholar
- Shadish, W. R., Cook, T. D., & Campbell, D. T. (2002). In W. R. Shedish, T. D. Cook, & D. T. Campbell (Eds.), Experimental and quasi-experimental designs for generalized causal inference. Boston, MA: Houghton Mifflin. Google Scholar
- Sherman, L. W., Gartin, P. R., & Buerger, M. E. (1989). Hot spots of predatory crime: Routine activities and the criminology of place. Criminology, 27(1), 27–56. ArticleGoogle Scholar
- Taxman, F. S. (1998). Reducing recidivism through a seamless system of care: Components of effective treatment, supervision, and transition services in the community. Washington, DC: Bureau of Governmental Research. Google Scholar
- Weisburd, D. (2000). Randomized experiments in criminal justice policy: Prospects and problems. Crime & Delinquency, 46(2), 181–193. ArticleGoogle Scholar
- Weisburd, D. (2005). Hot spots policing experiments and criminal justice research: Lessons from the field. The Annals of the American academy of political and social science, 599(1), 220–245. ArticleGoogle Scholar
- Weisburd, D., & Green, L. (1995). Policing drug hot spots: The Jersey City drug market analysis experiment. Justice Quarterly, 12(4), 711–735. https://doi.org/10.1080/07418829500096261.
- Weisburd, D., & Gill, C. (2014). Block randomized trials at places: Rethinking the limitations of small N experiments. Journal of Quantitative Criminology, 30(1), 97–112. ArticleGoogle Scholar
- Weisburd, D., Lum, C. M., & Petrosino, A. (2001). Does research design affect study outcomes in criminal justice? The Annals of the American Academy of Political and Social Science, 578(1), 50–70. ArticleGoogle Scholar
- Weisburd, D., Petrosino, A., & Fronius, T. (2013). Randomized experiments in criminology and criminal justice. In D. Weisburd & G. Bruinsma (Eds.), Encyclopedia of criminology and criminal justice. New York, NY: Springer Verlag. Google Scholar
- Weisburd, D., Wilson, D. B., & Mazerolle, L. (2020). Analyzing block randomized studies: The example of the Jersey City drug market analysis experiment. Journal of Experimental Criminology, 16(2), 265–287. ArticleGoogle Scholar
- Wilkinson, L. (1999). Statistical methods in psychology journals: Guidelines and explanations. American Psychologist, 54(8), 594–604. ArticleGoogle Scholar
- Wolraich, M. L., Wilson, D. B., & White, J. W. (1995). The effect of sugar on behavior or cognition in children: A meta-analysis. JAMA, 274(20), 1617–1621. ArticleGoogle Scholar
Author information
Authors and Affiliations
- Institute of Criminology, Faculty of Law, Hebrew University of Jerusalem, Hebrew University of Jerusalem Inst. of Criminology, Fac. of Law, Inst. of Criminology, Fac. of Law, Fairfax, VA, USA, Jerusalem, Israel David Weisburd
- Department of Criminology, Law and Society, George Mason University, Manassas, VA, USA David B. Wilson
- Department of Criminal Justice, Temple University, Philadelphia, PA, USA Alese Wooditch
- Department of Sociology, Iowa State University Department of Sociology, Ames, IA, USA Chester Britt
- David Weisburd